Hi all!
Welcome to the latest issue of your guide to AI, an editorialized newsletter covering key developments in AI research (particularly for this issue!), industry, geopolitics and startups during April 2023. Apologies for the delay in shipping this issue out - it’s been a wild time in AI lately…and we’ve now passed 22,000 subscribers, thank you for your readership! Before we kick off, a couple of news items from us :-)
Thanks to those of you who joined the NYC and SF LLM best practices meetups I hosted last month! You can read a tl;dr of the learnings here, courtesy of my friend Louis Coppey and watch this 1 min video to catch the SF meetup vibe.
On 23rd June in London we’re hosting the 7th Research and Applied AI Summit, featuring speakers from Intercom, Northvolt, Meta, Cruise, Roche/Genentech, Oxford and more. RSVP here as we’ll be issuing ticket links soon.
Careers @ Air Street: If you or someone in your network is particularly excited about developer/ML relations, leading our work in building AI communities, synthesising and distributing best practices, and building new AI-first products using the latest tools please reply here!
As usual, we love hearing what you’re up to and what’s on your mind, just hit reply or forward to your friends :-)
🌎 The (geo)politics of AI
It has now been 2 months since GPT-4 was released by OpenAI and usage figures of ChatGPT appear to be nothing short of spectacular. Meanwhile, calls for regulation, safeguards, and the protection of consumer data grow louder - increasingly with a focus on GPT-like models. President Biden remarked to his Council of Advisors on Science and Technology that “tech companies have a responsibility, in my view, to make sure their products are safe before making them public.” When asked “Mr. President, do you think AI is dangerous?” he replied “It remains to be seen. It could be.” In May, the CEOs of major US AI technology companies including Google, Microsoft, OpenAI, and Anthropic are due to meet the Vice President Kamala Harris to discuss AI and safety.
Weaving this together in a case to slow down AI race dynamics, my State of AI Report co-author Ian Hogarth penned a oped in the FT, We must slow down the race to God-like AI. It is certainly true, as per our 2021 report, that investments into model capabilities has vastly outstripped efforts in developing theory, controllability and thus alignment of the same systems. While I’m not in the camp that AGI will lead to the end of human life as we know it (or variations of this argument), I do believe that just as with any powerful technology, it is important to deliver it in a reliable, robust, and safe way. Invoking deities, loss of life and livelihood, isn’t a productive route to achieving a pragmatic outcome. For example, EU lawmakers are now drawing distinctions between foundation models and general purpose AI models (what is the difference, really?) when it comes to what system developers must or mustn’t produce to be able to market their solutions in Europe.
There is still so much that we have to learn about today’s systems. It turns out that LLMs might suffer from more fundamental alignment issues: “for any behavior that has a finite probability of being exhibited by the model, there exist prompts that can trigger the model into outputting this behavior, with probability that increases with the length of the prompt. This implies that any alignment process that attenuates undesired behavior but does not remove it altogether, is not safe against adversarial prompting attacks.”
In the UK, the government announced a £100M foundation model taskforce to “ensure sovereign capabilities and broad adoption of safe and reliable foundation models, helping cement the UK’s position as a science and technology superpower by 2030”. Let’s see what happens next, I’m not exactly sure. One of the topics I suspect they might cover is large-scale data licensing for model training. For example, could the BBC open its media archives for training in exchange for revenue? We held this prediction when creating last year’s State of AI Report - that content owners such as Reddit or Getty would turn to enterprise licenses for large scale training on their corpuses. Reddit is now moving in this direction.
Meanwhile, the Ministry of Electronics and Information Technology of India has confirmed that the government “is not considering bringing a law or regulating the growth of AI in the country.” Instead, India appears to be leaning into the upsides of enabling AI development: “the Government of India sees AI as a significant and strategic area for the country and technology sector. It further believes that AI will have kinetic effect for the growth of entrepreneurship & business and Government is taking all necessary steps in policies and infrastructure to develop a robust AI sector in the country.”
LLMs are also making their way into the defense sector. On the one hand, Palantir demonstrated the use of LLMs in their AI Platform as a battlefield assistant. The system could take instructions for commanding resources on the battlefield and process intelligence on classified networks and devices on the edge. Relatedly, a team from Marine Corps University and Scale AI tailored an LLM for military planning against an adversary. They were able to load the adversary’s doctrine, open source intelligence and literature on deterrence, and subsequently ask questions like “What is a joint blockade?” to “How does country X employ diesel submarines?”. Users felt that the system was “outstanding at helping students answer doctrine-related questions that assisted with the development of adversary courses of action.”
🏭 Big tech
Lots is being written now about moats in AI - which companies have them and where do they come from? First, a leaked blog post from Google outlining how their business (and OpenAI’s) has no moats in AI and that open source is of key importance. Statements like “directly competing with open source is a losing proposition” and “we need them more than they need us” set the tone…”Google should establish itself a leader in the open source community, taking the lead by cooperating with, rather than ignoring, the broader conversation. This probably means taking some uncomfortable steps, like publishing the model weights for small ULM variants. This necessarily means relinquishing some control over our models. But this compromise is inevitable. We cannot hope to both drive innovation and control it.”
My current take is to avoid prognosticating too strongly about where moats exist or don’t exist. Everything is moving too quickly (see Stanford’s Ecosystem Graphs that track models, datasets and applications), which leads to high-variance over the longer term. Secrets or tricks of the trade behind highly capable models are hard to keep under wraps in an environment that is particularly volatile - here, open source wins. Another take is that high-value (and usually higher-risk) tasks will birth their specialised AI models (and systems), while the longer tail of tasks can be served by the larger models. Instead, let’s focus back on the basis: find a user problem and bring a genuinely robust solution to bear. This is how Replit is approaching their code generation LLMs, which power their goal of helping anyone build software. Long term, my view is that one of the ultimate moats is “I won’t get fired for buying XYZ”. A business has to do whatever it takes to become XYZ, whether that means better technology, distribution, brand, pricing or else.
The other big news at Google was the merging of Google Brain (largely in SF) and DeepMind (largely in London) to (re)form Google DeepMind into a single entity led by Demis and with Jeff Dean taking on the role of Chief Scientist. Many in the field heralded this move as one that demonstrates the company’s seriousness in competing with OpenAI et al. I’d agree, particularly that more centralised, organised teams that are dedicated to a single goal tend to outcompete their peers (see: 5 years of GPT progress).
🍪 Hardware (kinda)
Self driving trials continue to push forward. In the UK, Wayve has begun automated delivery of groceries from Asda with a human safety driver behind the wheel on London’s busy streets. In SF, Cruise has been operating night-time drives for consumers with no safety drive for what feels like quite some time. They’re expanding the service to Houston and Dallas, and are pursuing an expanded zone in California. I took the service in late December 2022 and was honestly amazed at its quality (thanks, Oliver Cameron!). Last month, I took a daytime ride in Waymo and was equally impressed. Seriously, I do think self-driving services are here and will work despite all the downer press in the space. In fact, Oliver’s recent tweet thread on how self-driving has progressed in 7 years really shows the positive impact machine learning has had.
Meanwhile, Google published a technical report on its TPUv4 system, which benchmarks positively compared to NVIDIA’s A100 and Graphcore’s Bow. Elon’s X Corp (parent org that owns Twitter) purchased 10k NVIDIA GPUs to prime his AI effort.
🎁 What’s next?
It’s all about autonomous agents (AutoGPT, BabyAGI and others) on AI Twitter at the moment. These systems essentially chain together LLMs and use them as “agents” that can devise stepwise strategies for solving tasks and go ahead to execute them using tools. This is pretty much the dream of many who envision software automating repetitive tasks. While these open source libraries capture the imagination, I believe the devil is likely in the long(de)tail. I see this in my own experience, if a system that overpromises and under delivers within 1-2 tries, I lose trust and default back to my existing solution. One could say although autonomous agents reduce the requisite knowledge for building automated systems, they need to back that up with robustness and reliability out of the box. We’re not there yet, but I’m watching this space.
🔬Research
Segment Anything, Meta. The modern deep learning era was started by a successful image classification model (the now household name AlexNet). Computer vision continued to headline ML research (together with reinforcement learning) through impressive results on classification and segmentation until transformers came out in 2017 (not that computer vision research was stalling). Then language models, and more generally content generation became the big thing: GPT-2 showed transformers could generate grammatically correct sentences; GANs were hitting their stride; OpenAI built CLIP, which paved the way for text-to-image generation. Promptable language models, and their integration with image generation pipelines, made ML more appealing to a wider audience. But image classification and segmentation were seemingly left behind. Again, seemingly: A large part of the applications of deep learning in the industry came from image classification and segmentation. But not anymore. Meta introduced a large-scale project called “Segment Anything” which included the release of 1B segmentation masks on a 11M image dataset, as well as a segmentation model called SAM. As done in the article, the project can be summarized in three points:
The task definition: promptable segmentation. The goal of the project is to build a model that can segment an image given any text prompt, even if it doesn’t exist in the training dataset, i.e. zero-shot generalization.
The model: the model needs to support flexible prompts, compute masks in real-time for interactive use, and it needs to be ambiguity aware. The model architecture that satisfies these constraints turned out to be simple: they use an image encoder (a vision transformer – ViT) to compute image embeddings, a text encoder (the CLIP text encoder) for prompt embedding, and the combined embeddings are fed through a masked decoder that predicts segmentation masks. One benefit of having a separate image encoder is that the image embedding can be reused for other prompts. This is particularly important for interactive use where one will generally want to adjust their prompts to have better segmentation results. They handle ambiguity by training to predict multiple maps for the same prompt.
The dataset: unlike text, segmentation masks aren’t available en masse on the web. Building a segmentation dataset can quickly become very costly (ask any computer vision company hiring annotators to build their image segmentation dataset). To remedy this, the researchers developed a “data-engine” through a model-in-the-loop dataset annotation. The data engine has 3 stages of increasing automation: “(i) SAM assists annotators in segmentation, (ii) SAM automatically generates masks by prompting it with likely object locations and annotators focus on annotating the remaining objects, helping increase mask diversity, (iii) SAM is prompted with a regular grid of foreground points, yielding on average ∼100 high-quality masks per image”
Meta tested SAM on 23 segmentation tasks and reported a performance that is often only slightly below manually annotated images. Notably, they show that through prompt engineering, SAM can be used on other tasks including edge detection, object proposal generation, and instance segmentation. Meta released the SA-1B dataset for researchers and made SAM open-source. This is a truly remarkable project. Follow-ups (and concurrent work) are already underway:
Segment Everything Everywhere All at Once: concurrent work from University of Wisconsin-Madison, Microsoft, and HKUST.
Personalize Segment Anything Model with One Shot: allows users to better customize SAM for specific visual concepts by giving SAM an additional reference mask.
Track Anything: Segment Anything Meets Videos: combines SAM and XMem (a video object segmentation model) for live object tracking.
Now for our periodic checkup of how long the input of transformers can be:
Unlimiformer: Long-Range Transformers with Unlimited Length Input, Carnegie Mellon University. To avoid the quadratic dependency of the encoder-decoder models on their input, CMU researchers suggest (i) choosing a small desired context length, (ii) dividing a potentially long sequence into chunks of this length, (iii) storing each encoded chunk using an encoder in a datastore, (iv) at each decoding step, querying the datastore using a k-NN search. This is done efficiently through a smart reformulation of attention (check Section 2.3 of the paper if you’re curious about this). This allows the model to summarize up to 350k token-long inputs. A nice feature of their method is that it can be used on top of existing encoder-decoder models like LongFormer.
Scaling Transformer to 1M tokens and beyond with RMT, DeepPavlov, AIRI, LIMS. During training, sequences of up to 4096 tokens are divided into 7 segments of 512 tokens. Segments are sequentially fed through transformer blocks that are augmented with global memory tokens which are carried over to the next transformer block, as one would do with regular recurrent neural networks. During inference (where one doesn’t do memory and computation intensive backpropagation), the same process can be performed over longer sequences of 2M+ tokens. Read this too fast and you’d think the expensive long range dependence of transformers is solved. But what this paper actually does is to bring the power of transformers to recurrent neural networks. A strength of recurrent neural networks (when implemented correctly) is to not forget about early sequences. A strength of transformers is to improve language understanding of any sequence of words as long as it’s not too long (otherwise it becomes too expensive). This model will thus be able to identify segments where specific facts are and analyze them reasonably well, but it won’t be able to coherently use all the spanned text (the 2M+ tokens) to generate an answer.
Are Emergent Abilities of Large Language Models a Mirage? Stanford University. “Our alternative suggests that existing claims of emergent abilities are creations of the researcher’s analyses, not fundamental changes in model behavior on specific tasks with scale.” The authors present evidence that emergent abilities aren’t fundamental properties of scaling AI models. They argue that the sharp increase in LLM performance performance and its unpredictability is a result of “the researcher choosing a metric that nonlinearly or discontinuously deforms per-token error rates, and partially by possessing too few test data to accurately estimate the performance of smaller models (thereby causing smaller models to appear wholly unable to perform the task) and partially by evaluating too few large-scale models.”
Instruction Tuning with GPT-4, Microsoft. Take task T, use Giant Model instead of model M. Watch Giant Model crush every M on T. Here Giant Model = {GPT-4} and T = {generate instructions used to fine-tune language models}. Remember that fine tuning self-supervised language models with human-written instructions has been critical in training GPT-3.5 and other state of the art language models. And Stanford’s Alpaca (among others) showed how the instruction writing process can be delegated to very good LLMs. Microsoft researchers show here that “52K English and Chinese instruction-following data generated by GPT-4 lead to superior zero-shot performance on new tasks to the instruction-following data generated by previous state-of-the-art models.”
Synthetic Data from Diffusion Models Improves ImageNet Classification, Google Research. Now take Giant Model = {Google’s Imagen} and T = {Generate synthetic images to train models}. Generating synthetic images – and more generally synthetic data – to train computer vision models has been an early fantasy of ML researchers, but it’s now a concrete reality. Here, Google researchers show that large-scale text-to-image diffusion models can generate class-conditional images (i.e. images from a given label in a given dataset) that results in significant improvements in ImageNet classification accuracy over strong ResNet and Vision Transformer baselines trained solely on the real ImageNet.
Align your Latents: High-Resolution Video Synthesis with Latent Diffusion Models, NVIDIA. Since video synthesis is a particularly resource-intensive task, latent diffusion models, which train a diffusion model in latent space rather than in the pixel-space, are a natural fit. Here, researchers from NVIDIA use a latent diffusion model as a backbone image generation model, then they turn the image generator into a video generator by including a temporal dimension to the latent space diffusion model (LDM) and fine-tune it on videos. A temporal dimension is also added to the upsamplers (which go from the latent space to the pixel space) to recover actual images. It is possible to simply use pre-trained LDMs like Stable Diffusion and only train the temporal alignment model. As a result, they can generate short videos with resolution of up to 1280x2048. The sample quality is really impressive.
Also on our radar:
In-Context Learning Unlocked for Diffusion Models, UT Austin, Microsoft Azure AI. Given a pair of images (typically an input and the desired output) and text guidance, a model automatically understands the task and performs it on a new image query.
Learning Agile Soccer Skills for a Bipedal Robot with Deep Reinforcement Learning, DeepMind. Trained a humanoid robot with deep reinforcement learning to play simplified 1v1 soccer. TRaining was done in simulation and transferred to real robots zero-shot. Robots were optimized only for scoring, but ended up “walking 156 % faster, taking 63 % less time to get up, and kicking 24 % faster than a scripted baseline.”
Top-down design of protein architectures with reinforcement learning, University of Washington. Replaces bottom-up de novo design approaches with top-down RL to simulate evolutionary selection for function that naturally happened in multisubunit protein assemblies.
CodeGen2: Lessons for training LLMs on programming and natural languages, Salesforce Research. Release of the second generation of Salesforce’s open source programming language models.
HuggingGPT: Solving AI Tasks with ChatGPT and its Friends in Hugging Face, Zhejiang University and Microsoft Research Asia, and TaskMatrix.AI: Completing Tasks by Connecting Foundation Models with Millions of APIs, Microsoft. In these two papers, authors explore how an LLM can be used as a reasoning agent and controller on top of an ecosystem of more specialized models. A user would input a problem and the agent figures out how to solve it using a combination of said downstream models.
Blinded, randomized trial of sonographer versus AI cardiac function assessment, Stanford and Cedars Sinai. This clinical trial showed that AI assisted analysis of heart scan images saved clinicians time and the assessments generated by an AI were indistinguishable from those that weren’t.
Vicuna: An Open-Source Chatbot Impressing GPT-4 with 90%* ChatGPT Quality, UC Berkeley, UCSD, CMU, MBZUAI. This work develops a 13B parameter ChatGPT style system by fine-tuning a LLaMA base model with 70,000 user conversations with ChatGPT from sharegpt.com.
Generative Agents: Interactive Simulacra of Human Behavior, Stanford and Google. This work creates agents who learn to The Sims and operate in that world - they show how agents can simulate believable human-like behavior too.
Rethink reporting of evaluation results in AI, Cambridge and others. This piece correctly pushes the community to report more granular metrics, record all evaluation results, and create benchmarks that test specific capabilities.
De novo design of protein interactions with learned surface fingerprints, EPFL and Oxford. This work makes use of geometric deep learning to devise a “surface-centric approach” that captures the physical and chemical determinants of molecular recognition, enabling an approach for the de novo design of protein interactions and, more broadly, of artificial proteins with function.
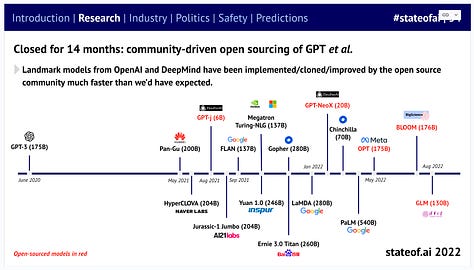
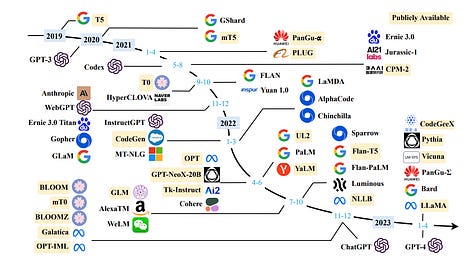
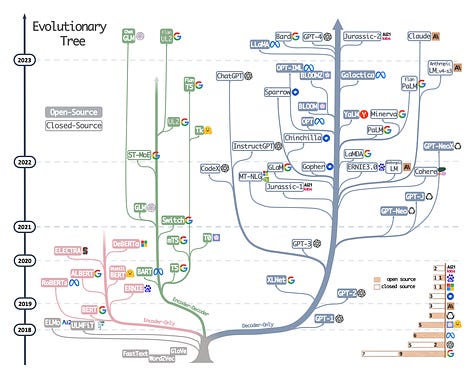
💰Startups
Funding highlight reel
LangChain, the popular open source framework for building LLM-based applications, raised a $10M Seed led by Benchmark followed by a $20-$25M Series A at a $200M valuation led by Sequoia a few months later.
Covariant, the e-commerce warehouse pick and place robot, expanded their Series C by another $75M led by existing investors on top of the $80M it raised in 2021.
Enveda, the drug discovery company deriving molecules from plants, expanded their Series B to $119M with additional capital from Kinnevik and the founder of KKR.
Harvey, an LLM-based legal software company, raised a $21M Series A led by Sequoia.
OpenAI completed a $300M share sale from investors including Sequoia, Andreessen Horowitz, Thrive and K2 Global at a $27-29B valuation.
Pinecone, one of the hot vector database companies, raised a $100M Series B at a $750M valuation.
Weaviate, another vector database company, raised a $50M Series B led by Index.
Replit, the collaborative software development and education tool, raised $97M at a $1.16B valuation led by a16z Growth to expand their AI capabilities (as discussed above!).
Veo Robotics, a robotics safety company enabling robots and humans to coexist in warehouses, raised a $29M Series B including capital from Amazon.
AlphaSense, the financial data company, raised a $100M round (on top of its previously announced $225M Series B) led by CapitalG to accelerate the integration of AI technologies.
Exits
Nothing particularly noteworthy of late! Far more investments :-)
---
Signing off,
Nathan Benaich, Othmane Sebbouh, 21 May 2023
Air Street Capital | Twitter | LinkedIn | State of AI Report | RAAIS | London.AI
Air Street Capital is a venture capital firm investing in AI-first technology and life science companies. We’re an experienced team of investors and founders based in Europe and the US with a shared passion for working with entrepreneurs from the very beginning of their company-building journey.
So interesting and helpful as always! Thank you so much for the information
Fantastic write up of the latest whirlwind developments in AI over the last month 🔥